End-to-end Model-based Gait Recognition
Xiang Li (Nanjing University of Science and Technology)*, Yasushi Makihara ("""Osaka University, Japan"""), Chi Xu (Nanjing University of Science and Technology), Yasushi Yagi (Osaka University), Shiqi Yu (Southern University of Science and Technology, China), Mingwu Ren (Nanjing University of Science and Technology)
Keywords: Biometrics
Abstract:
Most existing gait recognition approaches adopt a two-step procedure: a preprocessing step to extract silhouettes or skeletons followed by recognition. In this paper, we propose an end-to-end model-based gait recognition method. Specifically, we employ a skinned multi-person linear (SMPL) model for human modeling, and estimate its parameters using a pre-trained human mesh recovery (HMR) network. As the pre-trained HMR is not recognition-oriented, we fine-tune it in an end-to-end gait recognition framework. To cope with differences between gait datasets and those used for pre-training the HMR, we introduce a reconstruction loss between the silhouette masks in the gait datasets and the rendered silhouettes from the estimated SMPL model produced by a differentiable renderer. This enables us to adapt the HMR to the gait dataset without supervision using the ground-truth joint locations. Experimental results with the OU-MVLP and CASIA-B datasets demonstrate the state-of-the-art performance of the proposed method for both gait identification and verification scenarios, a direct consequence of the explicitly disentangled pose and shape features produced by the proposed end-to-end model-based framework.
SlidesLive
Similar Papers
MagGAN: High-Resolution Face Attribute Editing with Mask-Guided Generative Adversarial Network
Yi Wei (University at Albany - SUNY)*, Zhe Gan (Microsoft), Wenbo Li (Samsung Research America), Siwei Lyu (University at Albany), Ming-Ching Chang (University at Albany - SUNY), Lei Zhang (Microsoft), Jianfeng Gao (Microsoft Research), Pengchuan Zhang (Microsoft Research AI)
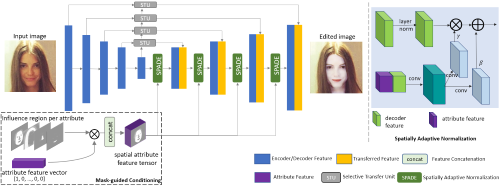
Domain-transferred Face Augmentation Network
Hao-Chiang Shao (Fu Jen Catholic University), Kang-Yu Liu (National Tsing Hua University), Chia-Wen Lin (National Tsing Hua University)*, Jiwen Lu (Tsinghua University)
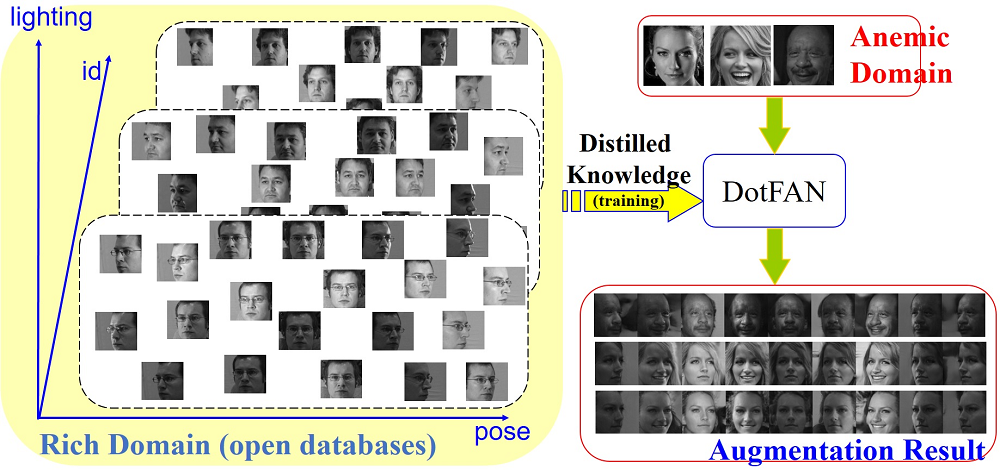
Learning 3D Face Reconstruction with a Pose Guidance Network
Pengpeng Liu (The Chinese University of Hong Kong)*, Xintong Han (Malong Technologies), Michael Lyu (The Chinese University of Hong Kong), Irwin King (The Chinese University of Hong Kong), Jia Xu (Huya AI)
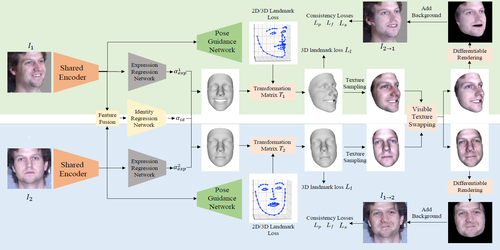