D2D: Keypoint Extraction with Describe to Detect Approach
Yurun Tian (Imperial College London)*, Vassileios Balntas (Scape Technologies), Tony Ng (Imperial College London), Axel Barroso-Laguna (Imperial College London), Yiannis Demiris (Imperial College London), Krystian Mikolajczyk (Imperial College London)
Keywords: Recognition: Feature Detection, Indexing, Matching, and Shape Representation
Abstract:
In this paper, we present a novel approach that exploits the information within the descriptor space to propose keypoint locations. Detect then describe, or detect and describe jointly are two typical strategies for extracting local descriptors. In contrast, we propose an approach that inverts this process by first describing and then detecting the keypoint locations. Describe-to-Detect (D2D) leverages successful descriptor models without the need for any additional training. Our method selects keypoints as salient locations with high information content which is defined by the descriptors rather than some independent operators. We perform experiments on multiple benchmarks including image matching, camera localisation, and 3D reconstruction. The results indicate that our method improves the matching performance of various descriptors and that it generalises across methods and tasks.
SlidesLive
Similar Papers
Descriptor-Free Multi-View Region Matching for Instance-Wise 3D Reconstruction
Takuma Doi (Osaka University), Fumio Okura (Osaka University)*, Toshiki Nagahara (Osaka University), Yasuyuki Matsushita (Osaka University), Yasushi Yagi (Osaka University)
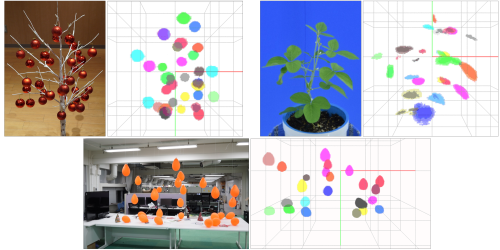
Modeling Cross-Modal interaction in a Multi-detector, Multi-modal Tracking Framework
Yiqi Zhong (University of Southern California)*, Suya You (US Army Research Laboratory), Ulrich Neumann (USC)
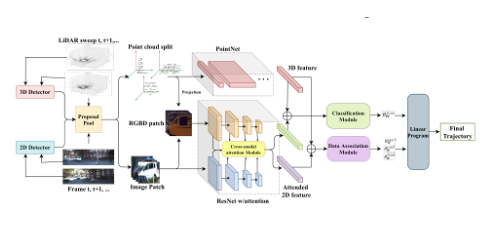
HDD-Net: Hybrid Detector Descriptor with Mutual Interactive Learning
Axel Barroso-Laguna (Imperial College London)*, Yannick Verdie (Huawei Noah's Ark Lab), Benjamin Busam (Technical University of Munich), Krystian Mikolajczyk (Imperial College London)
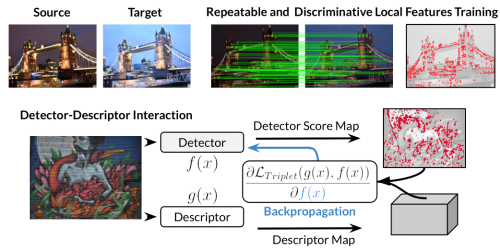