Betrayed by Motion: Camouflaged Object Discovery via Motion Segmentation
Hala Lamdouar (University of Oxford)*, Charig Yang (University of Oxford), Weidi Xie (University of Oxford), Andrew Zisserman (University of Oxford)
Keywords: Motion and Tracking
Abstract:
The objective of this paper is to design a computational architecture that discovers camouflaged objects in videos, specifically by exploiting motion information to perform object segmentation. We make the following three contributions: (i) We propose a novel architecture that consists of two essential components for breaking camouflage, namely, a differentiable registration module to align consecutive frames based on the background, which effectively emphasises the object boundary in the difference image, and a motion segmentation module with memory that discovers the moving objects, while maintaining the object permanence even when motion is absent at some point. (ii) We collect the first large-scale Moving Camouflaged Animals (MoCA) video dataset, which consists of over 140 clips across a diverse range of animals (67 categories). (iii) We demonstrate the effectiveness of the proposed model on MoCA, and achieve competitive performance on the unsupervised segmentation protocol on DAVIS2016 by only relying on motion.
SlidesLive
Similar Papers
Novel-View Human Action Synthesis
Mohamed Ilyes Lakhal (Queen Mary University of London)*, Davide Boscaini (Fondazione Bruno Kessler), Fabio Poiesi (Fondazione Bruno Kessler), Oswald Lanz (Fondazione Bruno Kessler, Italy), Andrea Cavallaro (Queen Mary University of London, UK)
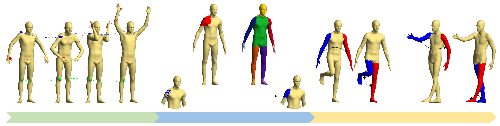
Reconstructing Creative Lego Models
George Tattersall (University of York)*, Dizhong Zhu (University of York), William A. P. Smith (University of York), Sebastian Deterding (University of York), Patrik Huber (University of York)
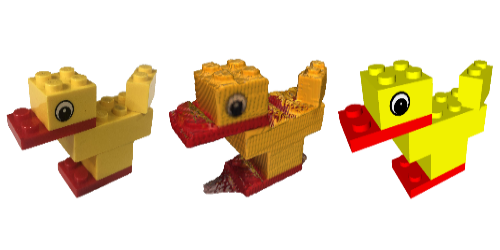
V2A - Vision to Action: Learning robotic arm actions based on vision and language
Michal Nazarczuk (Imperial College London)*, Krystian Mikolajczyk (Imperial College London)
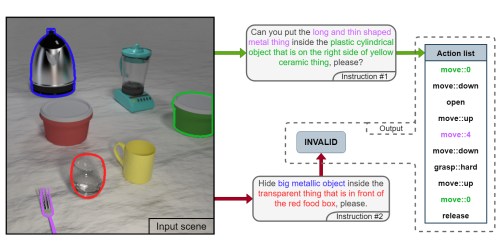