Learning End-to-End Action Interaction by Paired-Embedding Data Augmentation
Ziyang Song (Institute of Artificial Intelligence and Robotics, Xi'an Jiaotong University.)*, Zejian Yuan (Xi‘an Jiaotong University), Chong Zhang (Tencent Robotics X), Wanchao Chi (Tencent Robotics X), Yonggen Ling (Tencent), Shenghao Zhang (Tencent)
Keywords: Applications of Computer Vision, Vision for X
Abstract:
In recognition-based action interaction, robots' responses to human actions are often pre-designed according to recognized categories and thus stiff.In this paper, we specify a new Interactive Action Translation (IAT) task which aims to learn end-to-end action interaction from unlabeled interactive pairs, removing explicit action recognition.To enable learning on small-scale data, we propose a Paired-Embedding (PE) method for effective and reliable data augmentation.Specifically, our method first utilizes paired relationships to cluster individual actions in an embedding space.Then two actions originally paired can be replaced with other actions in their respective neighborhood, assembling into new pairs.An Act2Act network based on conditional GAN follows to learn from augmented data.Besides, IAT-test and IAT-train scores are specifically proposed for evaluating methods on our task.Experimental results on two datasets show impressive effects and broad application prospects of our method.
SlidesLive
Similar Papers
V2A - Vision to Action: Learning robotic arm actions based on vision and language
Michal Nazarczuk (Imperial College London)*, Krystian Mikolajczyk (Imperial College London)
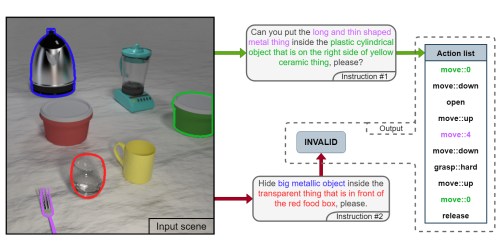
Long-Term Cloth-Changing Person Re-identification
Xuelin Qian (Fudan University), Wenxuan Wang (Fudan University), Li Zhang (University of Oxford), Fangrui Zhu (Fudan University), Yanwei Fu (Fudan University)*, Tao Xiang (University of Surrey), Yu-Gang Jiang (Fudan University), Xiangyang Xue (Fudan University)
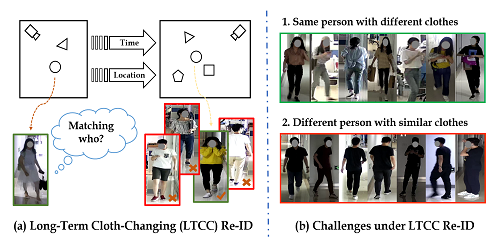
Novel-View Human Action Synthesis
Mohamed Ilyes Lakhal (Queen Mary University of London)*, Davide Boscaini (Fondazione Bruno Kessler), Fabio Poiesi (Fondazione Bruno Kessler), Oswald Lanz (Fondazione Bruno Kessler, Italy), Andrea Cavallaro (Queen Mary University of London, UK)
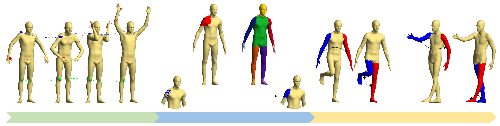