FKAConv: Feature-Kernel Alignment for Point Cloud Convolution
Alexandre Boulch (valeo.ai)*, Gilles Puy (Valeo), Renaud Marlet (Ecole des Ponts ParisTech)
Keywords: 3D Computer Vision
Abstract:
Recent state-of-the-art methods for point cloud semantic segmentation are based on convolution defined for point clouds The interest goes beyond semantic segmentation. We propose a formulation of the convolution for point cloud directly inspired by the discrete convolution in image processing. The resulting formulation underlines the separation between the discrete kernel space and the geometric space where the points lies. Several existing methods fall under this formulation.The two spaces are linked with a space change matrix $\mathbf{A}$, estimated with a neural network. $\mathbf{A}$ softly assigns the input features on the convolution kernel. Finally, we show competitive results on several semantic segmentation benchmarks while being efficient both in computation time and memory.
SlidesLive
Similar Papers
Sparse Convolutions on Continuous Domains for Point Cloud and Event Stream Networks
Dominic Jack (Queensland University of Technology)*, Frederic Maire (Queensland University of Technology), SIMON DENMAN (Queensland University of Technology, Australia), Anders Eriksson (University of Queensland )
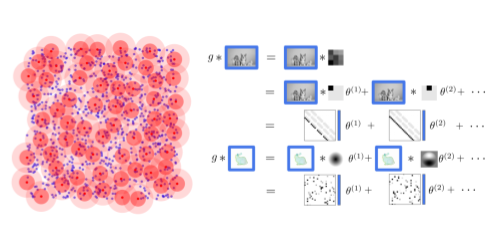
Progressive Batching for Efficient Non-linear Least Squares
Huu Le (Chalmers University of Technology)*, Christopher Zach (Chalmers University), Edward Rosten (Snap Inc.), Oliver J. Woodford (Snap Inc)
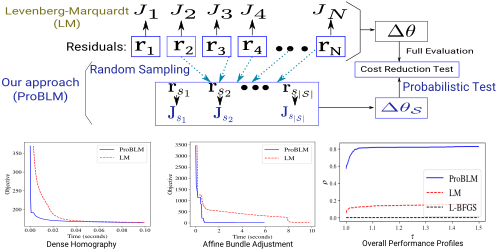
Encode the Unseen: Predictive Video Hashing for Scalable Mid-Stream Retrieval
Tong Yu (University of Strasbourg)*, Nicolas Padoy (University of Strasbourg)
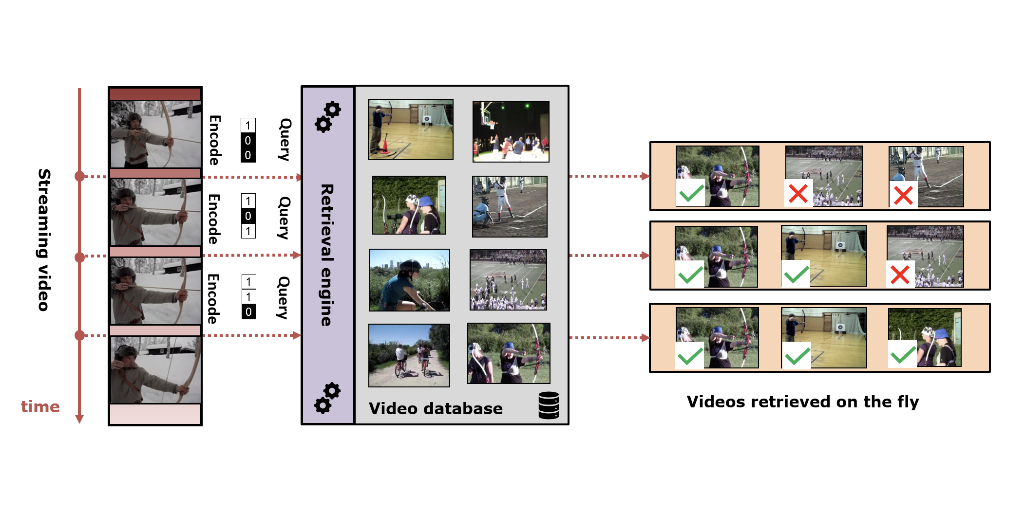