Deep Snapshot HDR Imaging Using Multi-Exposure Color Filter Array
Takeru Suda (Tokyo Institute of Technology), Masayuki Tanaka (Tokyo Institute of Technology), Yusuke Monno (Tokyo Institute of Technology)*, Masatoshi Okutomi (Tokyo Institute of Technology)
Keywords: Computational Photography, Sensing, and Display
Abstract:
In this paper, we propose a deep snapshot high dynamic range (HDR) imaging framework that can effectively reconstruct an HDR image from the RAW data captured using a multi-exposure color filter array (ME-CFA), which consists of a mosaic pattern of RGB filters with different exposure levels. To effectively learn the HDR image reconstruction network, we introduce the idea of luminance normalization that simultaneously enables effective loss computation and input data normalization by considering relative local contrasts in the "normalized-by-luminance'' HDR domain. This idea makes it possible to equally handle the errors in both bright and dark areas regardless of absolute luminance levels, which significantly improves the visual image quality in a tone-mapped domain. Experimental results using two public HDR image datasets demonstrate that our framework outperforms other snapshot methods and produces high-quality HDR images with fewer visual artifacts.
SlidesLive
Similar Papers
Chromatic Aberration Correction Using Cross-Channel Prior in Shearlet Domain
Kunyi Li (Tsinghua University), Xin Jin (Tsinghua University)*
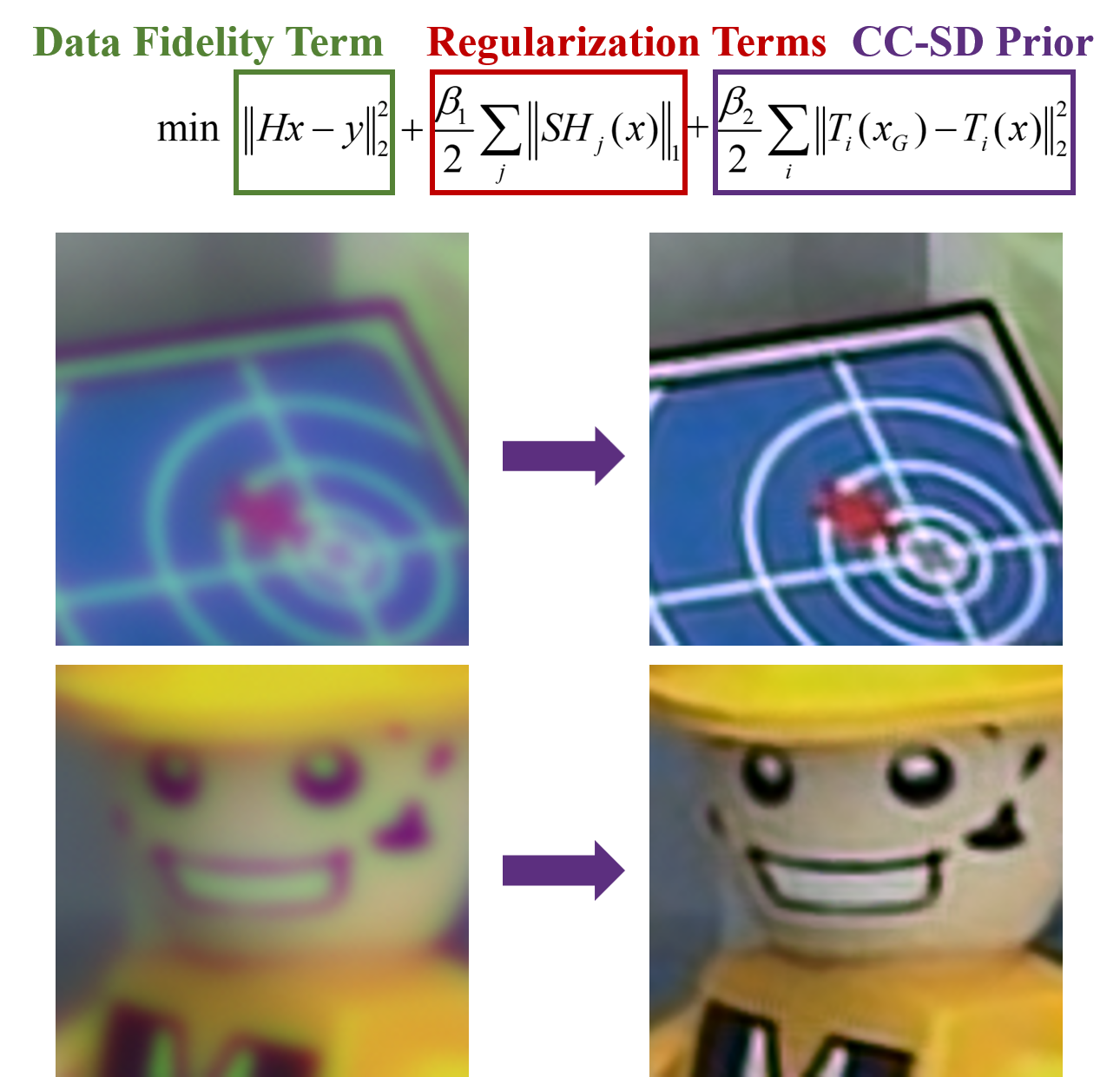
Frequency Attention Network: Blind Noise Removal for Real Images
Hongcheng Mo (Shanghai Jiao Tong University), Jianfei Jiang (Shanghai Jiao Tong University), Qin Wang (Shanghai Jiao Tong University)*, Dong Yin (Fullhan), Pengyu Dong (Fullhan), Jingjun Tian (Fullhan)
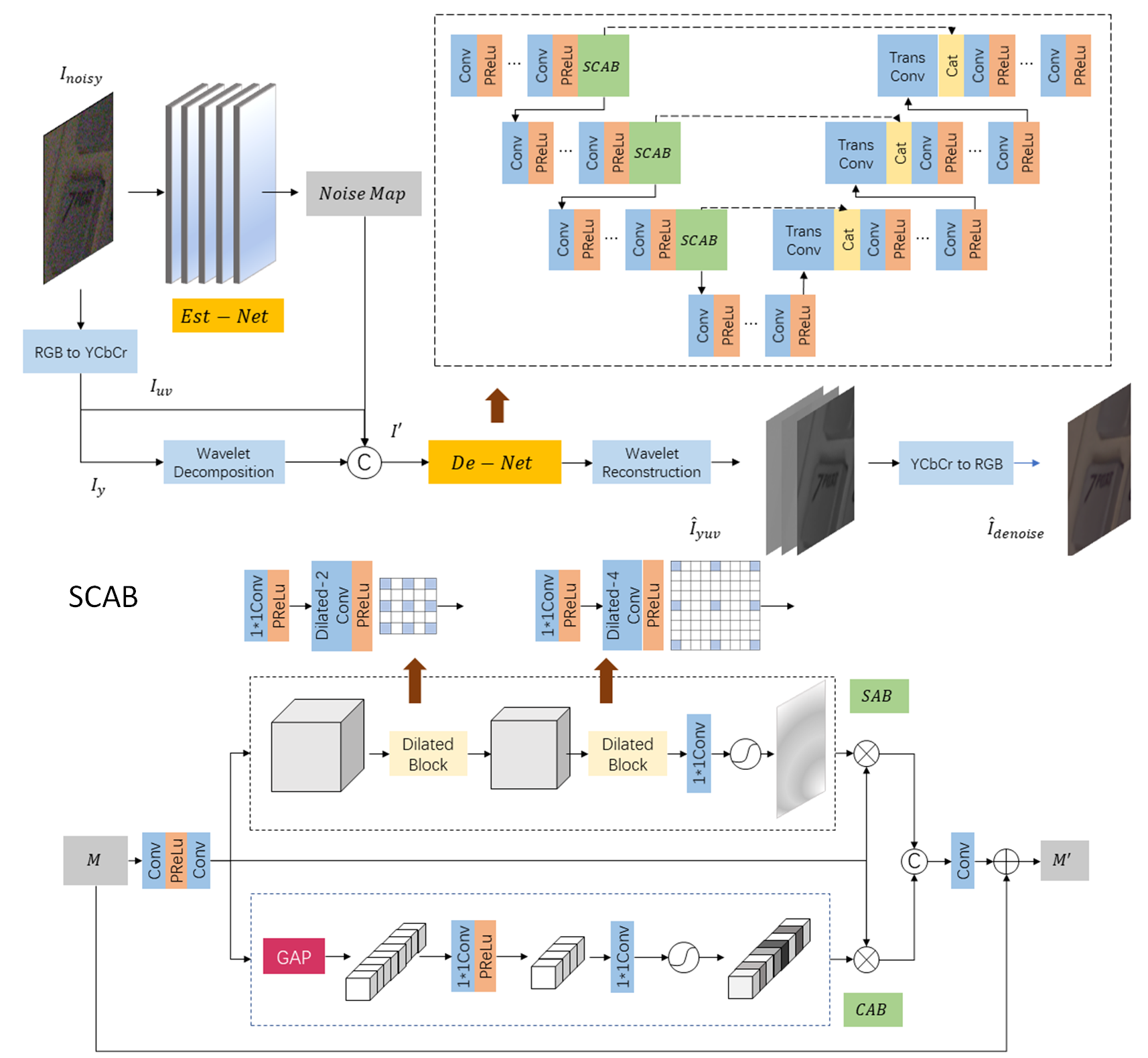
Dehazing Cost Volume for Deep Multi-view Stereo in Scattering Media
Yuki Fujimura (Kyoto University)*, Motoharu Sonogashira (Kyoto University), Masaaki Iiyama (Kyoto University)
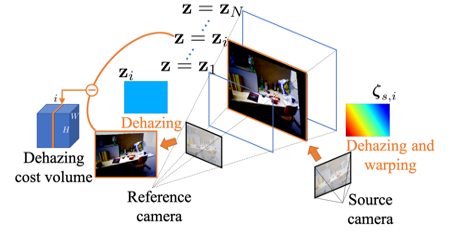