Uncertainty Estimation and Sample Selection for Crowd Counting
Viresh Ranjan (Stony Brook University)*, Boyu Wang (Stony Brook University), Mubarak Shah (University of Central Florida), Minh Hoai (Stony Brook University)
Keywords: Face, Pose, Action, and Gesture
Abstract:
We present a method for image-based crowd counting, one that can predict a crowd density map together with the uncertainty values pertaining to the predicted density map. To obtain prediction uncertainty, we model the crowd density values using Gaussian distributions and develop a convolutional neural network architecture to predict these distributions. A key advantage of our method over existing crowd counting methods is its ability to quantify the uncertainty of its predictions. We illustrate the benefits of knowing the prediction uncertainty by developing a method to reduce the human annotation effort needed to adapt counting networks to a new domain. We present sample selection strategies which make use of the density and uncertainty of predictions from the networks trained on one domain to select the informative images from a target domain of interest to acquire human annotation. We show that our sample selection strategy drastically reduces the amount of labeled data from the target domain needed to adapt a counting network trained on a source domain to the target domain. Empirically, the networks trained on the UCF-QNRF dataset can be adapted to surpass the performance of the previous state-of-the-art results on NWPU dataset and Shanghaitech dataset using only 17% of the labeled training samples from the target domain. Github Page: https://github.com/cvlab-stonybrook/UncertaintyCrowdCounting
SlidesLive
Similar Papers
GAN-based Noise Model for Denoising Real Images
Linh Duy Tran (Teikyo University)*, Son Minh Nguyen (Teikyo University), Masayuki Arai (Teikyo Univ.)
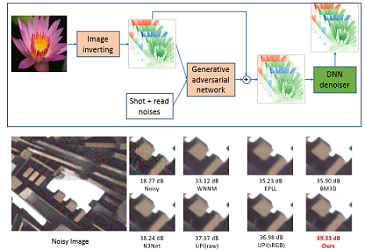
Pose Correction Algorithm for Relative Frames between Keyframes in SLAM
Youngseok Jang (Seoul National University)*, Hojoon Shin (Seoul National University), H. Jin Kim (Seoul National University)
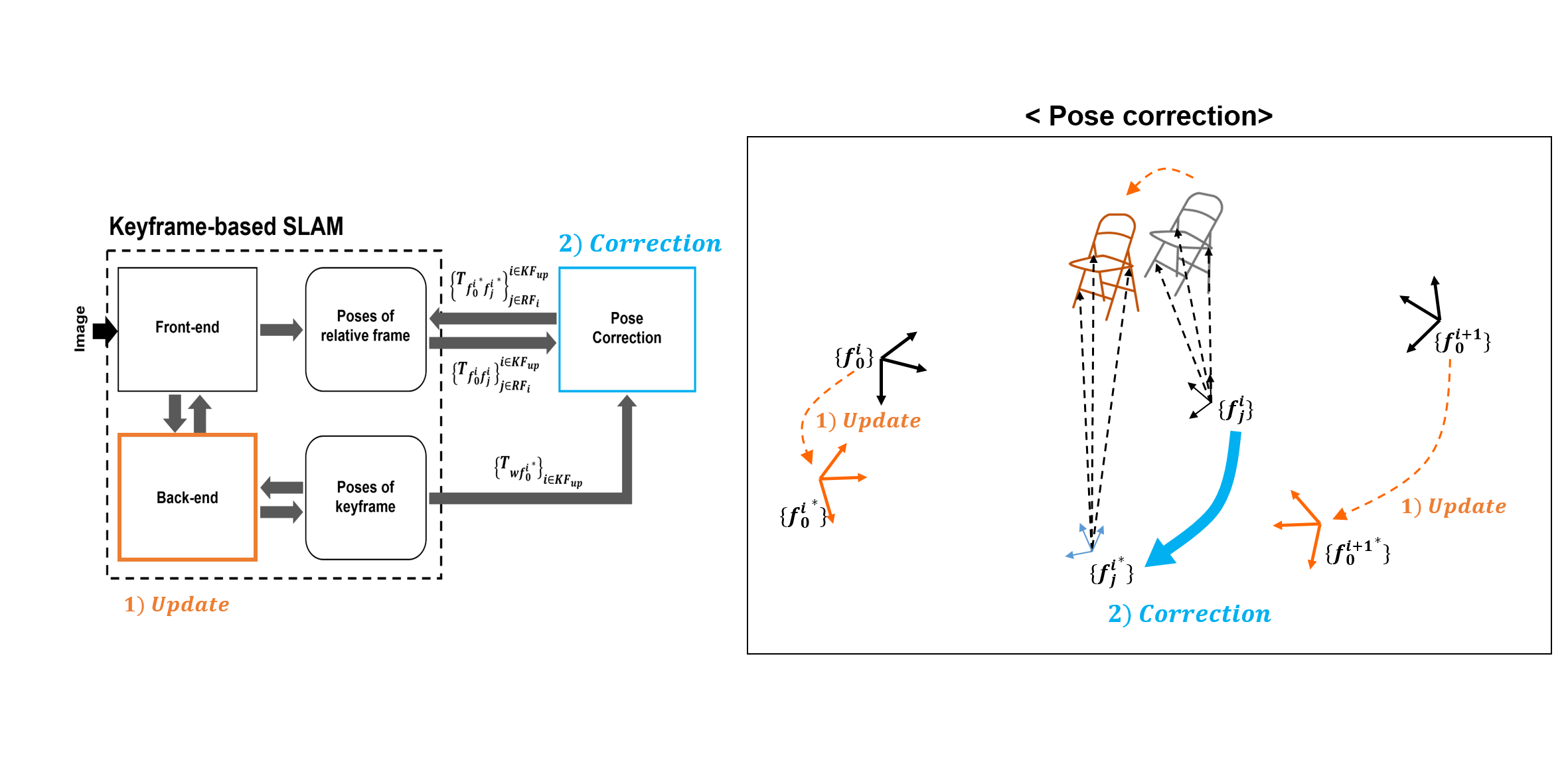
Channel Pruning for Accelerating Convolutional Neural Networks via Wasserstein Metric
Haoran Duan (University of Science and Technology of China (USTC))*, Hui Li (University of Science and Technology of China (USTC))
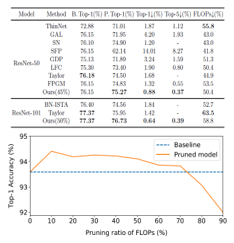