Local Context Attention for Salient Object Segmentation
Jing Tan (Megvii(face++) Research), Pengfei Xiong (Megvii(face++) Research)*, Zhengyi Lv (Megvii(face++) Research), Kuntao Xiao (Megvii(face++) Research), Yuwen He (Megvii(face++) Research)
Keywords: Segmentation and Grouping
Abstract:
Salient object segmentation aims at distinguishing various salient objects from backgrounds. Despite the lack of semantic consistency, salient objects often have obvious texture and location characteristics in local area. Based on this priori, we propose a novel Local Context Attention Network (LCANet) to generate locally reinforcement feature maps in a uniform representational architecture. The proposed network introduces an Attentional Correlation Filter (ACF) module to generate explicit local attention by calculating the correlation feature map between coarse prediction and global context. Then it is expanded to a Local Context Block(LCB). Furthermore, a one-stage coarse-to-fine structure is implemented based on LCB to adaptively enhance the local context description ability. Comprehensive experiments are conducted on several salient object segmentation datasets, demonstrating the superior performance of the proposed LCANet against the state-of-the-art methods, especially with 0.883 max F-score and 0.034 MAE on DUTS-TE dataset.
SlidesLive
Similar Papers
Spatial Class Distribution Shift in Unsupervised Domain Adaptation: Local Alignment Comes to Rescue
Safa Cicek (UCLA)*, Ning Xu (Adobe Research), Zhaowen Wang (Adobe Research), Hailin Jin (Adobe Research), Stefano Soatto (UCLA)
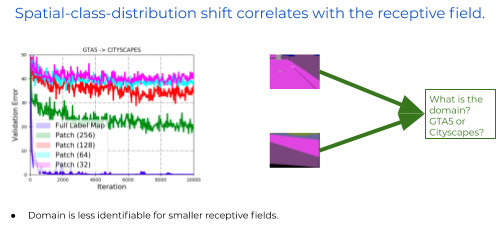
Visual Tracking by TridentAlign and Context Embedding
Janghoon Choi (Seoul National University)*, Junseok Kwon (Chung-Ang Univ., Korea), Kyoung Mu Lee (Seoul National University)
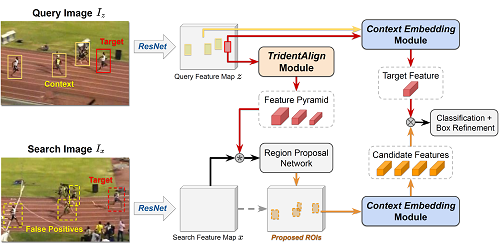
In-sample Contrastive Learning and Consistent Attention for Weakly Supervised Object Localization
Minsong Ki (Yonsei University)*, Youngjung Uh (Yonsei University), Wonyoung Lee (Yonsei University), Hyeran Byun (Yonsei University)
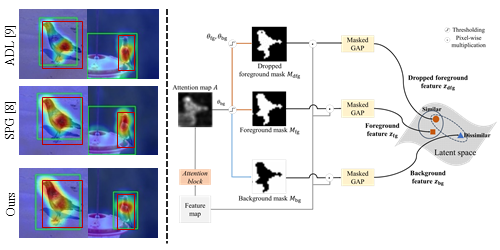