Spatial Class Distribution Shift in Unsupervised Domain Adaptation: Local Alignment Comes to Rescue
Safa Cicek (UCLA)*, Ning Xu (Adobe Research), Zhaowen Wang (Adobe Research), Hailin Jin (Adobe Research), Stefano Soatto (UCLA)
Keywords: Statistical Methods and Learning
Abstract:
We propose a method for semantic segmentation in unsupervised domain adaptation (UDA) setting. We particularly examine the domain gap between spatial-class distributions and propose to align the local distributions of the segmentation predictions. Despite its simplicity, the proposed method achieves state-of-the-art results in UDA segmentation benchmarks.
SlidesLive
Similar Papers
Local Context Attention for Salient Object Segmentation
Jing Tan (Megvii(face++) Research), Pengfei Xiong (Megvii(face++) Research)*, Zhengyi Lv (Megvii(face++) Research), Kuntao Xiao (Megvii(face++) Research), Yuwen He (Megvii(face++) Research)
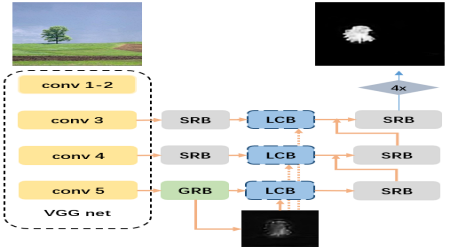
In-sample Contrastive Learning and Consistent Attention for Weakly Supervised Object Localization
Minsong Ki (Yonsei University)*, Youngjung Uh (Yonsei University), Wonyoung Lee (Yonsei University), Hyeran Byun (Yonsei University)
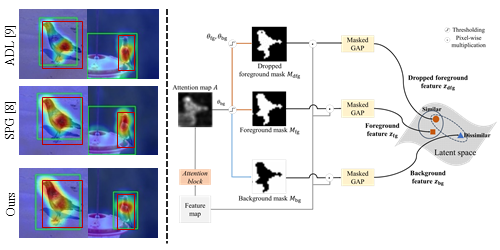
Local Facial Makeup Transfer via Disentangled Representation
Zhaoyang Sun (Wuhan University of Technology)*, Feng Liu (Wuhan University of Technology), Wen Liu (Wuhan University of Technology), Shengwu Xiong (Wuhan University of Technology), Wenxuan Liu (Wuhan University of Technology)
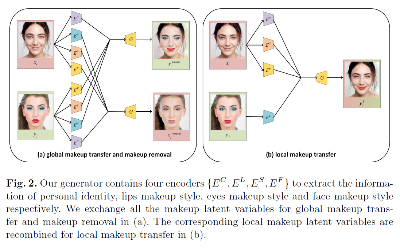