Attention-Based Fine-Grained Classification of Bone Marrow Cells
Weining Wang (South China University of Technology), Peirong Guo (South China University of Technology)*, Lemin Li (South China University of Technology), Yan Tan (Peking University People's Hospital), Hongxia Shi (Peking University People's Hospital), Yan Wei (Peking University People's Hospital), Xiangmin Xu (South China University of Technology)
Keywords: Biomedical Image Analysis
Abstract:
Computer aided fine-grained classification of bone marrow cells is a significant task because manual morphological examination is time-consuming and highly dependent on the expert knowledge. Limited methods are proposed for the fine-grained classification of bone marrow cells. This can be partially attributed to challenges of insufficient data, high intra-class and low inter-class variances.In this work, we design a novel framework Attention-based Suppression and Attention-based Enhancement Net (ASAE-Net) to better distinguish different classes. Concretely, inspired by recent advances of weakly supervised learning, we develop an Attention-based Suppression and Attention-based Enhancement (ASAE) layer to capture subtle differences between cells. In ASAE layer, two parallel modules with no training parameters improve the discrimination in two different ways. Furthermore, we propose a Gradient-boosting Maximum-Minimum Cross Entropy (GMMCE) loss to reduce the confusion between subclasses. In order to decrease the intra-class variance, we adjust the hue in a simple way. In addition, we adopt a balanced sampler aiming to alleviate the issue of the data imbalance.Extensive experiments prove the effectiveness of our method. Our approach achieves favorable performance against other methods on our dataset.
SlidesLive
Similar Papers
Self-Guided Multiple Instance Learning forWeakly Supervised Thoracic DiseaseClassification and Localizationin Chest Radiographs
Constantin Seibold (Karlsruhe Institute of Technology)*, Jens Kleesiek (German Cancer Research Center), Heinz-Peter Schlemmer (German Cancer Research Center), Rainer Stiefelhagen (Karlsruhe Institute of Technology)
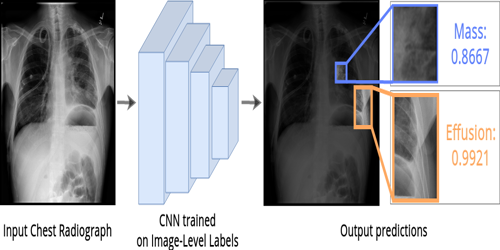
Hierarchical X-Ray Report Generation via Pathology tags and Multi Head Attention
Preethi Srinivasan (IIT Mandi), Daksh Thapar (Indian Institute of Technology, Mandi)*, Arnav Bhavsar (IIT Mandi), Aditya Nigam (IIT mandi)
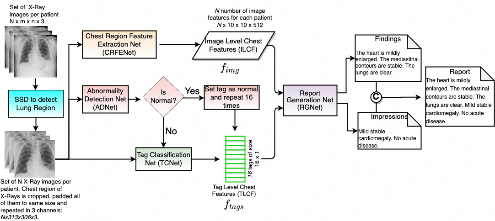
FootNet: An efficient convolutional network for multiview 3D foot reconstruction
Felix Kok (Cambridge University)*, James Charles (Cambridge University), Roberto Cipolla (University of Cambridge)
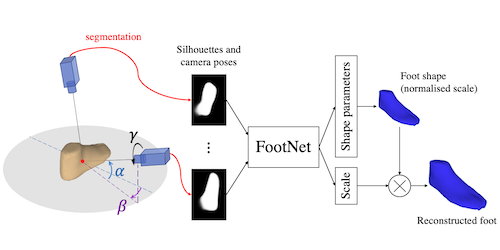