Scale-Aware Polar Representation for Arbitrarily-Shaped Text Detection
Yanguang Bi (SenseTime Research), Zhiqiang Hu (SenseTime Research)*
Keywords: Recognition: Feature Detection, Indexing, Matching, and Shape Representation
Abstract:
Arbitrarily-shaped text detection faces two major challenges: 1) various scales and 2) irregular angles. Previous works regress the text boundary in Cartesian coordinates as ordinary object detection. However, such grid space interleaves the unique scale and angle attributes of text, which seriously affects detection performance. The implicit disregard of text scale also impairs multi-scale detection ability. To better learn the arbitrary text boundary and handle the text scale variation, we propose a novel Scale-Aware Polar Representation (SAPR) framework. The text boundary is represented in Polar coordinates, where scale and angle of text could be both clearly expressed for targeted learning. This simple but effective transformation brings significant performance improvement. The explicit learning on separated text scale also promotes the multi-scale detection ability. Based on the Polar representation, we design line IoU loss and symmetry sine loss to better optimize the scale and angle of text with a multi-path decoder architecture. Furthermore, an accurate center line calculation is proposed to guide text boundary restoration under various scales. Overall, the proposed SAPR framework is able to effectively detect arbitrarily-shaped texts and tackle the scale variation simultaneously. The state-of-the-art results on multiple benchmarks solidly demonstrate the effectiveness and superiority of SAPR.
SlidesLive
Similar Papers
Unsupervised Domain Adaptive Object Detection using Forward-Backward Cyclic Adaptation
Siqi Yang (University of Queensland)*, Lin Wu (University of Queensland), Arnold Wiliem (the University of Queensland), Brian C. Lovell (University of Queensland)
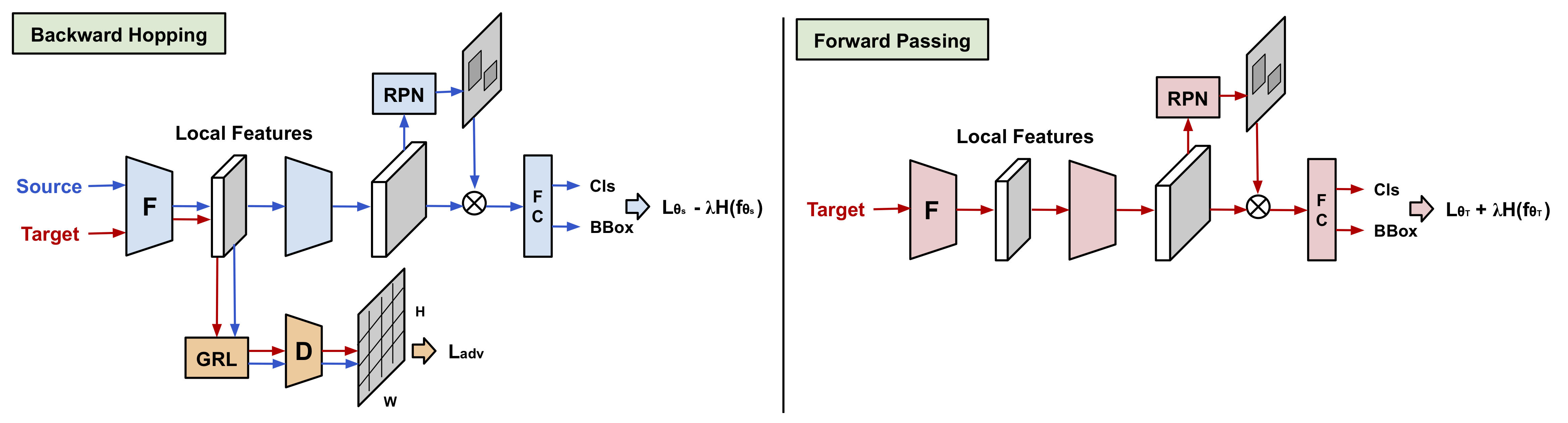
Synthetic-to-Real Unsupervised Domain Adaptation for Scene Text Detection in the Wild
weijia wu (Zhejiang University)*, Ning Lu (Tencent Cloud Product Department), Enze Xie (The University of Hong Kong), Yuxing Wang (Zhejiang University), Wenwen Yu (Xuzhou Medical University), Cheng Yang (Zhejiang University), HONG ZHOU (Zhejiang University)
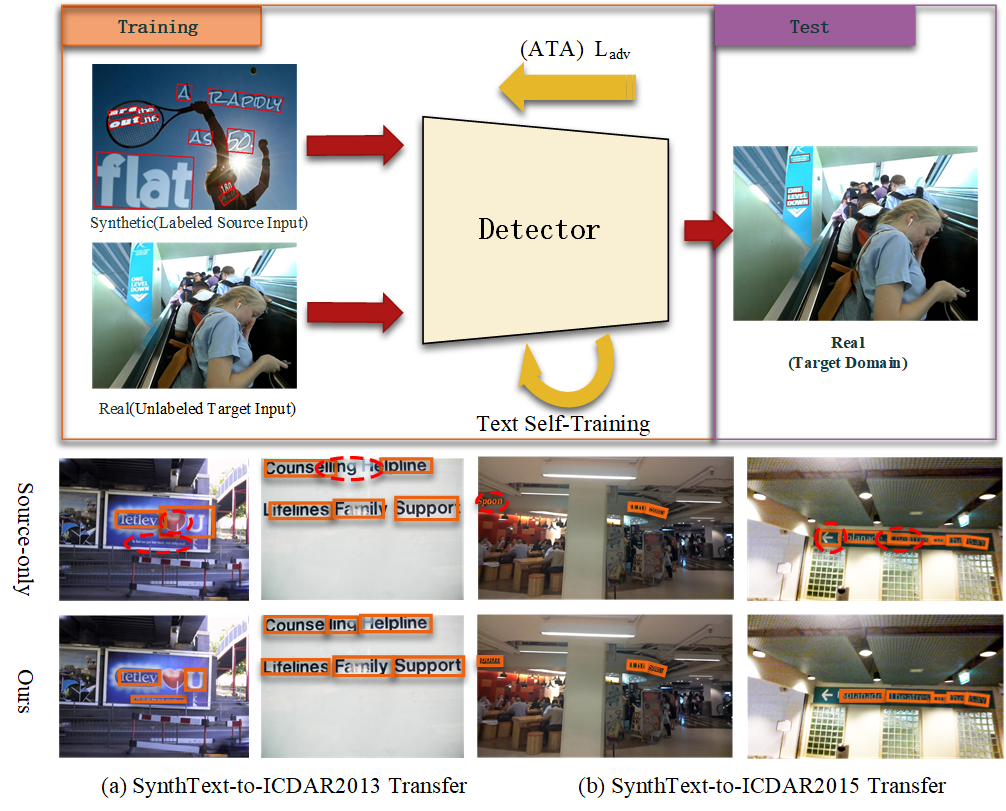
Domain Adaptation Gaze Estimation by Embedding with Prediction Consistency
Zidong Guo (Xi'an Jiaotong university)*, Zejian Yuan (Xi‘an Jiaotong University), Chong Zhang (Tencent Robotics X), Wanchao Chi (Tencent Robotics X), Yonggen Ling (Tencent), shenghao zhang (Tencent)
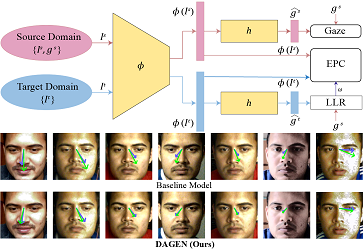