L2R GAN: LiDAR-to-Radar Translation
LeiChen Wang (Daimler AG)*, Bastian Goldluecke (University of Konstanz), Carsten Anklam (Daimler AG)
Keywords: Robot Vision
Abstract:
The lack of annotated public radar datasets causes difficulties for research in environmental perception from radar observations. In this paper, we propose a novel neural network based framework which we call L2R GAN to generate the radar spectrum of natural scenes from a given LiDAR point cloud.We adapt ideas from existing image-to-image translation GAN frameworks, which we investigate as a baseline for translating radar spectra image from a given LiDAR bird’s eye view (BEV). However, for our application, we identify several shortcomings of existing approaches. As a remedy, we learn radar data generation with an occupancy-grid-mask as a guidance, and further design a set of local region generators and discriminator networks. This allows our L2R GAN to combine the advantages of global image features and local region detail, and not only learn the cross-modal relations between LiDAR and radar in large scale, but also refine details in small scale. Qualitative and quantitative comparison show that L2R GAN outperforms previous GAN architectures with respect to details by a large margin. A L2R-GAN-based GUI also allows users to define and generate radar data of special emergency scenarios to test corresponding ADAS applications such as Pedestrian Collision Warning (PCW).
SlidesLive
Similar Papers
Overwater Image Dehazing via Cycle-Consistent Generative Adversarial Network
Shunyuan Zheng (Harbin Institute of Technology)*, Jiamin Sun (Harbin Institute of Technology), Qinglin Liu (Harbin Institute of Technology), Yuankai Qi (Harbin Institute of Technology), Shengping Zhang (Harbin Institute of Technology)
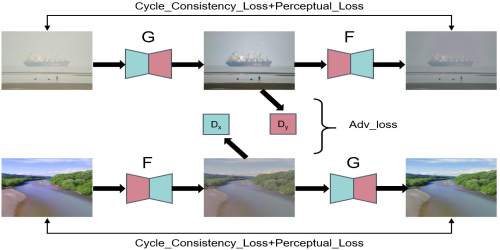
Semantics through Time: Semi-supervised Segmentation of Aerial Videos with Iterative Label Propagation
Alina Marcu (University "Politehnica" of Bucharest)*, Vlad Licaret (Autonomous Systems), Dragos Costea (University "Politehnica" of Bucharest), Marius Leordeanu (University "Politehnica" of Bucharest)
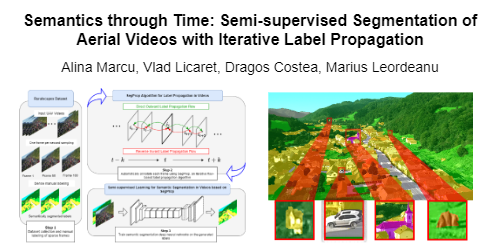
Multi-scale Attentive Residual Dense Network for Single Image Rain Removal
Xiang Chen (Shenyang Aerospace University ), Yufeng Huang (Shenyang Aerospace University)*, Lei Xu (Shenyang Fire Science and Technology Research Institute of MEM)
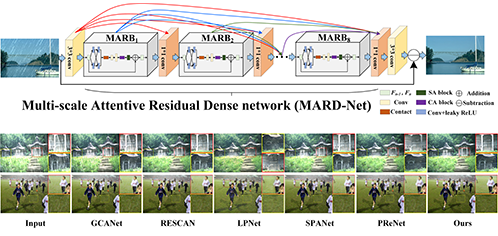