AFN: Attentional Feedback Network based 3D Terrain Super-Resolution
Ashish Kubade (International Institute Of Information Technology, Hyderabad)*, Diptiben Patel (IIIT Hyderabad), Avinash Sharma (CVIT, IIIT-Hyderabad), K. S. Rajan (IIIT Hyderabad)
Keywords: 3D Computer Vision
Abstract:
Terrain, representing features of an earth surface, plays a crucial role in many applications such as simulations, route planning, analysis of surface dynamics, computer graphics-based games, entertainment, films, to name a few. With recent advancements in digital technology, these applications demand the presence of high resolution details in the terrain. In this paper, we propose a novel fully convolutional neural network based super-resolution architecture to increase the resolution of low-resolution Digital Elevation Model (LRDEM) with the help of information extracted from the corresponding aerial image as a complementary modality. We perform the super-resolution of LRDEM using an attention based feedback mechanism named ‘Attentional Feedback Network�_(AFN), which selectively fuses the information from LRDEM and aerial image to enhance and infuse the high-frequency features and to produce the terrain realistically . We compare the proposed architecture with existing state-of-the-art DEM super-resolution methods and show that the proposed architecture outperforms enhancing the resolution of input LRDEM accurately and in a realistic manner.
SlidesLive
Similar Papers
TTPLA: An Aerial-Image Dataset for Detectionand Segmentation of Transmission Towers and Power Lines
Rabab Abdelfattah (University of South Carolina)*, XIAOFENG Wang (USC), Song Wang (University of South Carolina)
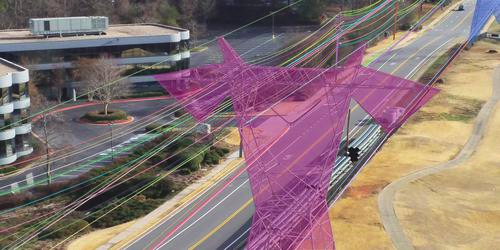
RGB-T Crowd Counting from Drone: A Benchmark and MMCCN Network
Tao Peng (Tianjin University), Qing Li (Northwestern Polytechnical University), Pengfei Zhu (Tianjin university)*
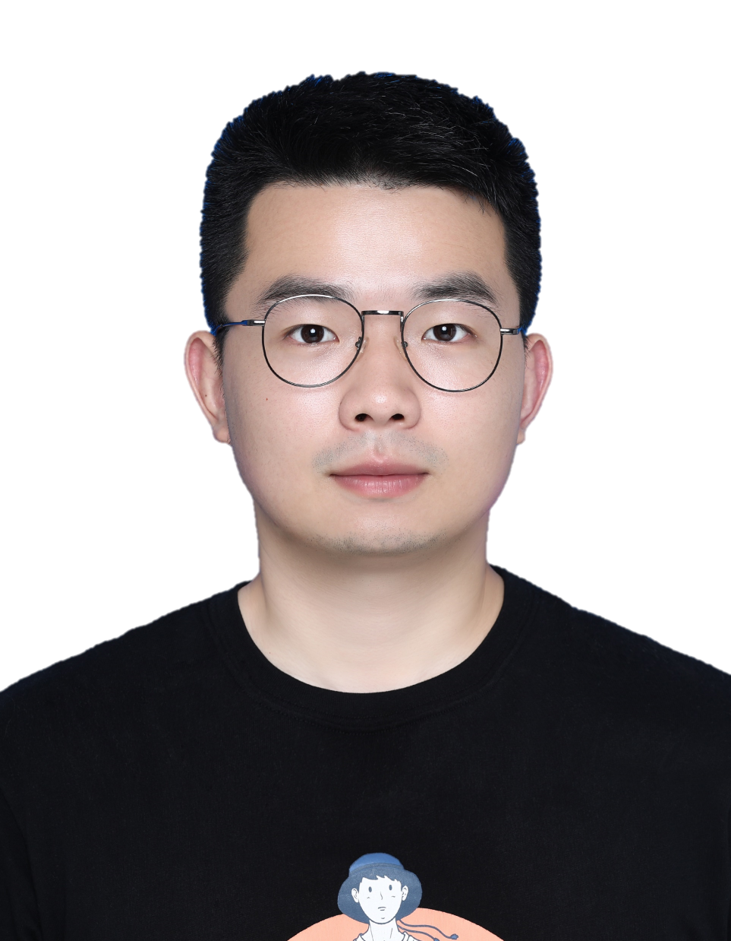
A Calibration Method for the Generalized Imaging Model with Uncertain Calibration Target Coordinates
David Uhlig (Karlsruhe Institute of Technology)*, Michael Heizmann (Karlsruher Institut fuer Technologie)
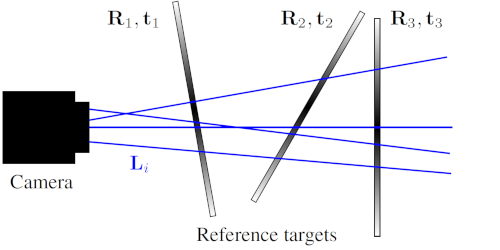