MCGKT-Net: Multi-level Context Gating Knowledge Transfer Network for Single Image Deraining
Kohei Yamamichi (Yamaguchi University)*, Xian-Hua Han (Yamaguchi University)
Keywords: Low-level Vision, Image Processing
Abstract:
Rain streak removal in a single image is very challenging task due to its ill-pose nature in essence. Recently, the end-to-end learning techniques with deep convolutional neural networks(DCNN) have made great progress in this task. However, the conventional DCNN-based deraining methods have struggled to exploit deeper and more complex network architectures for pursuing better performance. This study proposes a novel MCGKT-Net for boosting deraining performance, which is naturally multi-scale learning framework being capable of exploring multi-scale attributes of rain streaks and different semantic structures of the clear images. In order to obtain high representative features inside MCGKT-Net, we explore internal knowledge transfer module using ConvLSTM unit for conducting interaction learning between different layers, and investigate external knowledge transfer module for leveraging the knowledge already learned in other task domains. Furthermore, to dynamically select useful features in learning procedure, we propose a multi-scale context gating module in the MCGKT-Net using squeeze-and-excitation block. Experiments on three benchmark datasets: Rain100H, Rain100L and Rain800, manifest impressive performance compared with state-of-the-art methods.
SlidesLive
Similar Papers
Multi-scale Attentive Residual Dense Network for Single Image Rain Removal
Xiang Chen (Shenyang Aerospace University ), Yufeng Huang (Shenyang Aerospace University)*, Lei Xu (Shenyang Fire Science and Technology Research Institute of MEM)
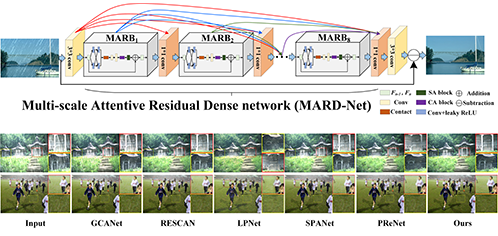
Overwater Image Dehazing via Cycle-Consistent Generative Adversarial Network
Shunyuan Zheng (Harbin Institute of Technology)*, Jiamin Sun (Harbin Institute of Technology), Qinglin Liu (Harbin Institute of Technology), Yuankai Qi (Harbin Institute of Technology), Shengping Zhang (Harbin Institute of Technology)
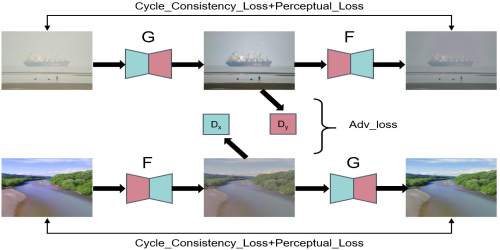
Vax-a-Net: Training-time Defence Against Adversarial Patch Attacks
Thomas Gittings (University of Surrey), Steve Schneider (University of Surrey), John Collomosse (Adobe Research)*
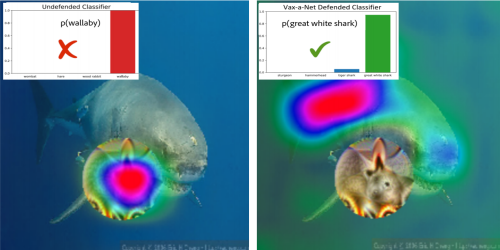