TSI: Temporal Scale Invariant Network for Action Proposal Generation
Shuming Liu (Shanghai Jiao Tong University), Xu Zhao (Shanghai Jiao Tong University)*, Haisheng Su (Shanghai Jiao Tong University), Zhilan Hu (Huawei)
Keywords: Video Analysis and Event Recognition
Abstract:
Despite the great progress in temporal action proposal generation, most state-of-the-art methods ignore the impact of action scales and the performance of short actions is still far from satisfaction. In this paper, we first analyze the sample imbalance issue in action proposal generation, and correspondingly devise a novel scale-invariant loss function to alleviate the insufficient learning of short actions. To further achieve proposal generation task, we adopt the pipeline of boundary evaluation and proposal completeness regression, and propose the Temporal Scale Invariant network. To better leverage the temporal context, boundary evaluation module generates action boundaries with high-precision-assured global branch and high-recall-assured local branch. Simultaneously, the proposal evaluation module is supervised with introduced scale-invariant loss, predicting accurate proposal completeness for different scales of actions. Comprehensive experiments are conducted on ActivityNet-1.3 and THUMOS14 benchmarks, where TSI achieves state-of-the-art performance. Especially, AUC performance of short actions is boosted from 36.53\% to 39.63\% compared with baseline.
SlidesLive
Similar Papers
SAUM: Symmetry-Aware Upsampling Module for Consistent Point Cloud Completion
Hyeontae Son (Seoul National University)*, Young Min Kim (Seoul National University)
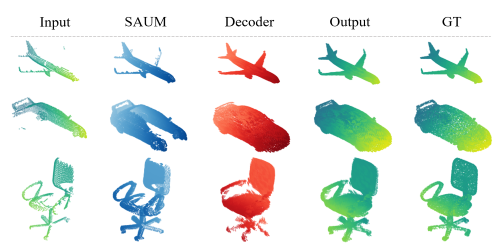
Reweighted Non-convex Non-smooth Rank Minimization based Spectral Clustering on Grassmann Manifold
Xinglin Piao (Peng Cheng Laboratory, Peking University, Dalian University of Technology)*, Yongli Hu (Beijing University of Technology), Junbin Gao (University of Sydney, Australia), Yanfeng Sun (Beijing University of Technology), Xin Yang (Dalian University of Technology), Baocai Yin (Beijing University of Technology)
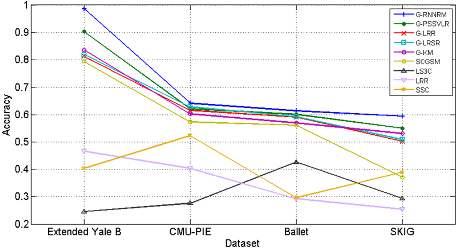
Mapping of Sparse 3D Data using Alternating Projection
Siddhant Ranade (University of Utah), Xin Yu (University of Utah), Shantnu Kakkar (Trimble), Pedro Miraldo (Instituto Superior Técnico, Lisboa), Srikumar Ramalingam (University of Utah)*
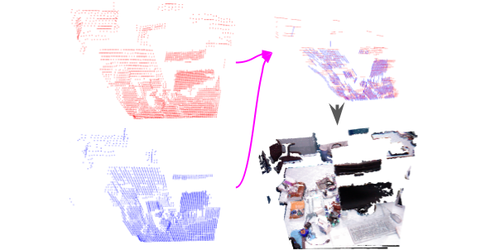