Backbone Based Feature Enhancement for Object Detection
Haoqin Ji (Shenzhen University), Weizeng Lu (Shenzhen University), Linlin Shen (Shenzhen University)*
Keywords: Recognition: Feature Detection, Indexing, Matching, and Shape Representation
Abstract:
FPN (Feature Pyramid Networks) and many of its variants have been widely used in state of the art object detectors and made remarkable progress in detection performance. However, almost all the architectures of feature pyramid are manually designed, which requires ad hoc design and prior knowledge. Meanwhile, existing methods focus on exploring more appropriate connections to generate features with strong semantics features from inherent pyramidal hierarchy of deep ConvNets (Convolutional Networks). In this paper, we propose a simple but effective approach, named BBFE (Backbone Based Feature Enhancement), to directly enhance the semantics of shallow features from backbone ConvNets. The proposed BBFE consists of two components: reusing backbone weight and personalized feature enhancement. We also proposed a fast version of BBFE, named Fast-BBFE, to achieve better trade-off between efficiency and accuracy. Without bells and whistles, our BBFE improves different baseline methods (both anchor-based and anchor-free) by a large margin (∼2.0 points higher AP) on COCO, surpassing common feature pyramid networks including FPN and PANet.
SlidesLive
Similar Papers
EPSNet: Efficient Panoptic Segmentation Network with Cross-layer Attention Fusion
Chia-Yuan Chang (National Taiwan University)*, Shuo-En Chang (National Taiwan University), Pei-Yung Hsiao (National University of Kaohsiung), Li-Chen Fu (National Taiwan University)
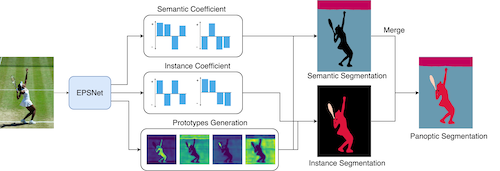
Bidirectional Pyramid Networks for Semantic Segmentation
Dong Nie (UNC)*, Jia Xue (Rutgers University), Xiaofeng Ren (Alibaba group)
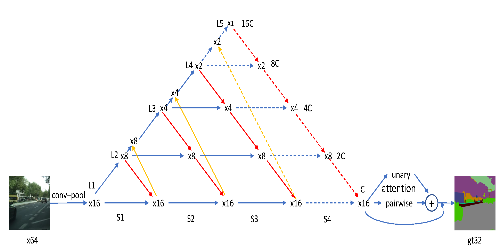
Best Buddies Registration for Point Clouds
Amnon Drory (Tel-Aviv University)*, Tal Shomer (Tel-Aviv University), Shai Avidan (Tel Aviv University), Raja Giryes (Tel Aviv University)
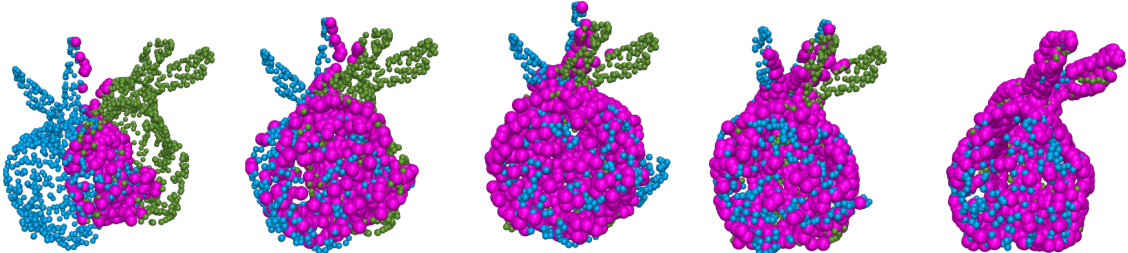