CLASS: Cross-Level Attention and Supervision for Salient Objects Detection
Lv Tang (Nanjing University)*, Bo Li (Nanjing University)
Keywords: Recognition: Feature Detection, Indexing, Matching, and Shape Representation; Segmentation and Grouping
Abstract:
Salient object detection (SOD) is a fundamental computer vision task. Recently, with the revival of deep neural networks, SOD has made great progresses. However, there still exist two thorny issues that cannot be well addressed by existing methods, indistinguishable regions and complex structures. To address these two issues, in this paper we propose a novel deep network for accurate SOD, named CLASS. First, in order to leverage the di_�erent advantages of low-level and high-level features, we propose a novel non-local cross-level attention (CLA), which can capture the long-range feature dependencies to enhance the distinction of complete salient object. Second, a novel cross-level supervision (CLS) is designed to learn complementary context for complex structures through pixel-level, region-level and object-level. Then the _�ne structures and boundaries of salient objects can be well restored. In experiments, with the proposed CLA and CLS, our CLASS net consistently outperforms 13 state-of-the-art methods on _�ve datasets.
SlidesLive
Similar Papers
SDCNet: Size Divide and Conquer Network for Salient Object Detection
Senbo Yan (Zhejiang University), Xiaowen Song (Zhejiang University)*, chuer yu (ZheJiang University)
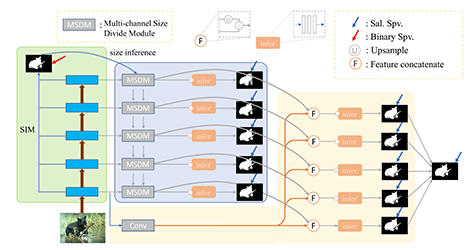
Branch Interaction Network for Person Re-identification
Zengming Tang (Shanghai Advanced Research Institute, Chinese Academy of Sciences, Shanghai, China)*, Jun Huang (Shanghai Advanced Research Institute, Chinese Academy of Sciences)
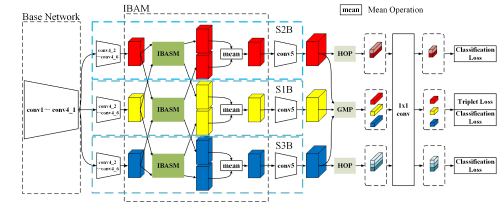
Generic Image Segmentation in Fully Convolutional Networks by Superpixel Merging Map
Jin-Yu Huang (National Taiwan University), Jian-Jiun Ding (National Taiwan University)*
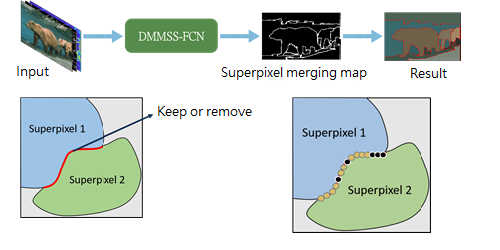